Erkan Basar
Computer scientist with a focus on artificial intelligence and language technologies
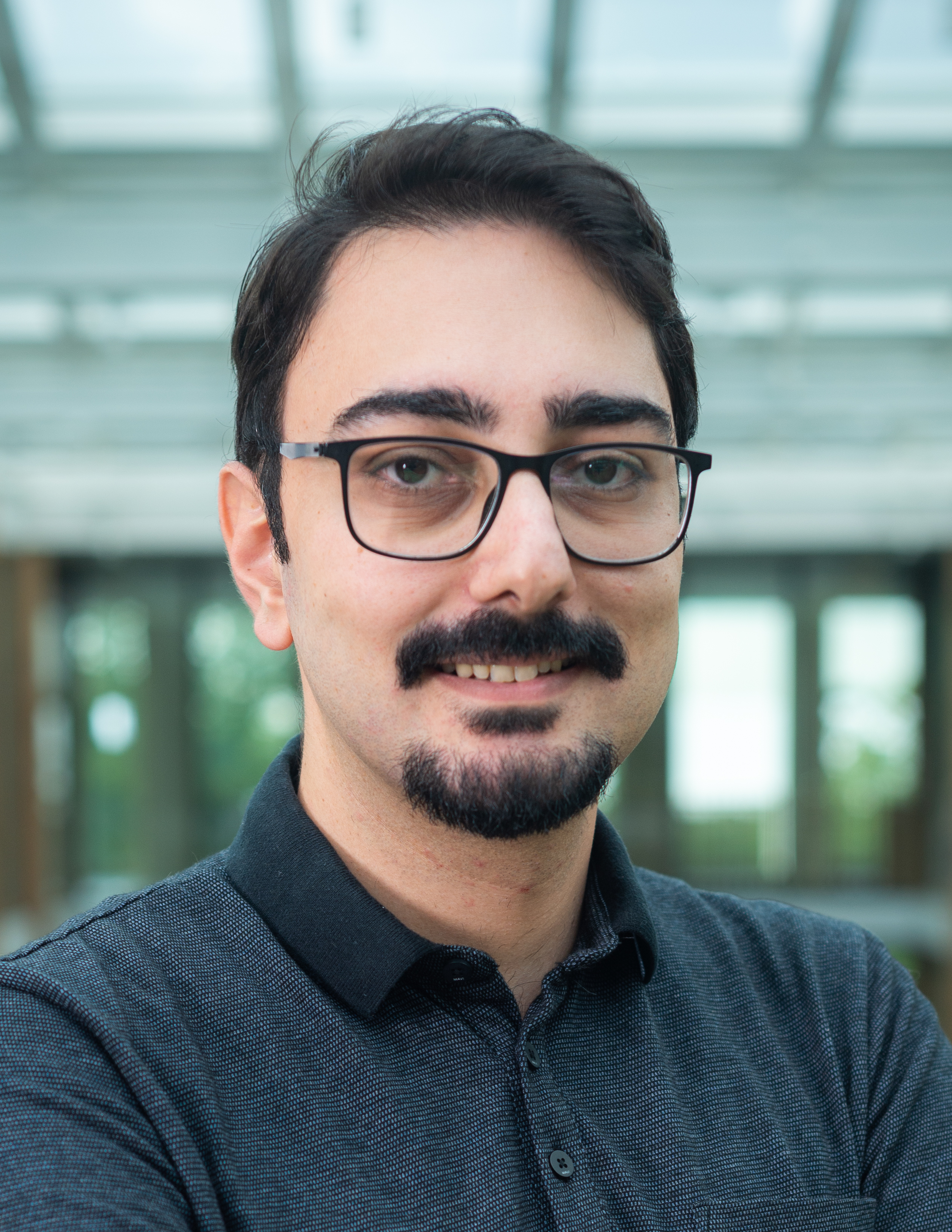
PhD Candidate
Radboud University
Nijmegen, Netherlands
I am a computer scientist specializing in AI and machine learning, with a focus on language technologies. My work is aimed at advancing research in AI and its practical applications. I enjoy working in multidisciplinary environments, collaborating with experts across various domains to bring innovative and effective solutions to complex challenges.
Currently, I work as a PhD candidate in Behavioural Science Institute at Radboud University. In my research, I focus on developing and evaluating intelligent conversational agents that support health counselling by using natural language processing methods and technologies, including large language models, as part of the multidisciplinary Look Who’s Talking project.
I obtained my master’s degree from Radboud University in Artificial Intelligence, and my bachelor’s degree from Muğla Sıtkı Koçman University in Computer Engineering. Shortly after, I have worked as an AI engineer and computational linguist at FloodTags where I have developed information systems that process textual data to collect critical information about natural disaster events in real-time from online social and mainstream media.
Recent News
Jan 21, 2025 | Publication and Presentation at COLING2025 |
---|---|
Dec 10, 2024 | Organizing Chatbots for Health Symposium |
Oct 19, 2024 | Invited Talk at BNAIC2024 |
Aug 15, 2024 | Publication and Poster at HCLLM at ACL2024 |
Jun 26, 2024 | Invited Talk at RadboudUMC Research Strategy Day |